The Food and Agriculture Organization of the United Nations estimates that by 2050, world food production needs to grow by 70% to feed a projected population of 9.1 billion people.
At the same time, income growth for farmers has been shrinking for decades and is almost certain to continue that trend.
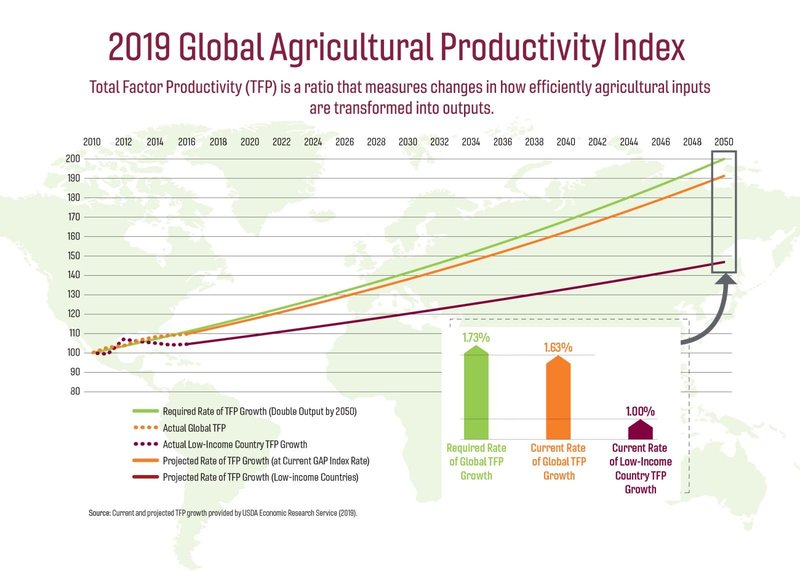
Source: New report says accelerating global agricultural productivity growth is critical
In order to raise production to meet demand, efficiency will be key. While there have been and will continue to be many innovations at the farm level (vertical farming and hydroponics come to mind), it is obvious that technology and software will play a crucial role in unlocking the necessary efficiency to meet demands at both a logistical and economic level.
It won't be easy though, and as we've built more and more software in the Agriculture industry here at Lofty, patterns are emerging that signal key challenges digital solutions will have to overcome in order to be effective.
1. Implement Data Fragmentation
The data coming off of farming implements has exploded in recent years and valuable insights are readily available once the data is properly analyzed. But, the data is extremely fragmented, making analytics a bigger integration problem than implementation problem. That is to say: it will be far easier to outfit the farm with machines that collect useful data than it will be to aggregate that data across equipment and gather insights.
Manufacturers like John Deere and Case collect and store data in proprietary formats that must be decoded into a common format where the data can be pivoted and shared. There are reasons, I suspect heavily economic, on why that is the case–the politics of which I don't intend to address here. The point is that in order to make use of this data, there is a critical need for digital products and software to automate this processing in order to do useful work with the information.
Standards like ESRI Shapefiles, and more recently GeoJSON and GeoPackages, aim to provide open standards for how this data is interchanged. Most implements do not output data directly to these formats though, so digital middleware is a necessary component to working with implement data.
2. Engineering for Data Volume
"Big data" is a term that has, thankfully, lost its charm over the past couple of years. I say thankfully because the term buried the nuanced challenges and varied solutions to the problem. I've written in the past on the definition of Big Data, which I believe is "Data that is larger than an organization has the widespread capability to support." For some organizations, this is billions of data points per day. For others, it's more data than can fit in an Excel spreadsheet.
Regardless, data volume is a large digital challenge. Many implements sample data at extremely high resolutions, like a data point for every 6 inches of implement travel. This precision is fantastic for doing analytics that is very statistically sound, but in practice isn't really useful to resolve whatever issues the data highlights.
Applicators, for example, lack the precision to apply a fertilizer mix that changes every 6 inches and many farms continue to rely on flown-on applications with small aircraft as a cost effective way of applying their fields. So, in order to make use of that huge volume of precise data, it must be stored raw and post-processed in order to aggregate into feasible activities that can be put to use in the field. For some farms, this might be at a field level, or more sophisticated operations might be able to subdivide fields into problem areas that require specific ratios of applicants.
We cannot expect farms to develop the expertise to perform Google-like levels of data engineering, so commercially available digital products have to fill the gap.
3. Productizing Machine Learning / AI
If data volume is massive and must be processed to refine its value, there is an implicit need for automation. In the field of Computer Science, it has become abundantly clear that Machine Learning techniques are the most viable solution to automating the large scale processing of vast amounts of data. For the uninitiated, you can think of Machine Learning as classes of algorithms that successfully shortcut the brute force approach to calculating information. It's a branch of Artificial Intelligence because Machine Learning is almost a computer assisted type of intuition. Don't get me wrong, there is heavy math involved and well trained algorithms can produce precise results, but they do so without exhaustively exploring all of the data in a set and rely heavily on volume and principles of statistics.
There is a tremendous amount of work going on in this field and the results will be powerful. Taking a step back from Agriculture for a moment, Machine Learning applications have a broad general challenge that will affect the farm as much as anywhere else: Taking these algorithms from Research and Development and actually operationalizing a usable product is difficult at best.
The skillsets required for the hard science of ML and the math-meets-creative world of software design are not necessarily interchangeable. The leap from a working algorithm on a Data Scientist's computer to a product that can support hundreds or thousands of concurrent operations is massive, and the process is fraught with both engineering and design challenges. We also must keep the end user in mind here–creating a Machine Learning tool that does not require a trained statistician to operate it requires the thoughtful coordination of data scientists, user experience designers, software engineers, data engineers, and product managers.
4. Solving Data Collection Bandwidth Constraints
All of the valuable data collected at the farm is bottlenecked by an infrastructure problem: internet bandwidth. It's no surprise that crop production is a distinctly rural enterprise, and it's common knowledge that rural internet access leaves much to be desired. In order for implement data to be processed, is must be co-located with the computational infrastructure that does the processing. The value of cloud based software is well documented (it can be accessed from anywhere, and shared with the click of a button), but the reason we must centralize data is, again, more of a logistical need than a user experience one. The reality is that there are many places on Earth where dropping a hard drive in the mail is more efficient than uploading on a weak 3G signal.
While internet access will continue to expand, and 5G networks will roll out higher bandwidth to more remote areas, there's no reason to believe that the volume of data won't simply grow to meet the capabilities of our infrastructure. Rural areas will continue to be disproportionally affected by this differential.
This is a place where concepts like Edge Computing will take center stage. We cannot expect the average farm to stand up and maintain a data center for its processing, but defeating bandwidth limitations is best done by colocating data storage and processing. Edge Computing stands to solve these types of problems by enabling cloud-like infrastructure to be deployed in the field on commodity hardware. You can think of this as a way of distributing the processing power of a datacenter to the individual farms, where local data can be stored and processed and only the harvested insights shipped to the cloud. Large enterprises like Chick-fil-a have made tech headlines recently for rolling out this kind of processing, which used to be centralized offsite, onto appliances that live in-store. The software can still be administered and updated remotely while continuing to provide the promise of reduced complexity and ease of access we've come to love about cloud computing.
5. Imagery-based Insights
At the leading edge of computing and Machine Learning research is computer vision. The same technology that is rapidly unlocking the feasibility of self driving cars stands to offer much potential to Agriculture. This science is still in its infancy, but to whatever extent aerial imagery can harvest useful data, it will be highly efficient compared to traditional data collection means.
Combine the very real notion that imagery (and other telemetry like LIDAR) can tell farmers something meaningful (elevation, slope, weed prevalence, pest prevalence are all being tested in the market) with the proliferation of commercial drone technology, and you can be assured that this data is far more efficiently collected from the air than the ground. It's not hard to imagine how much faster a drone can canvas a farm than a tractor implement, and at a substantially lower fuel cost.
This technology has a long way to go, but is already showing immense promise. Like other Machine Learning applications, though, there remains the tremendous difficulty of actually operationalizing products and services built on the underlying math and science.
It cannot be overstated that technology alone will not solve the world's food production needs. Solving socio-economic (incomes are shrinking and generational farming is waning), climate change, and land use challenges all must play a part. Technology is poised to be a key driver of efficiency which will be a multiplier on success in these other areas.
At Lofty, we're excited to have had the opportunity to do our small part in bringing some of this change to reality and we're eager to do more. The problems will be difficult, but the reward of making an impact will be fulfilling, and now more than ever, it's necessary.